Who We Are
Welcome to HKUST Vision and System Design Lab. The focus of our lab includes design, optimization and compression of artificial intelligence (AI) models, as well as architecture and design of AI chips/systems for energy-efficient training and inference of such AI models. Currently, we place strong focus on multimodal large foundation models for computer vision, vision-language, and medical applications. Our Lab also collaborates closely with InnoHK AI Chip Center for Smart Emerging Systems on co-design and co-optimization of AI systems across application, algorithm and hardware layers.
News
Highlights
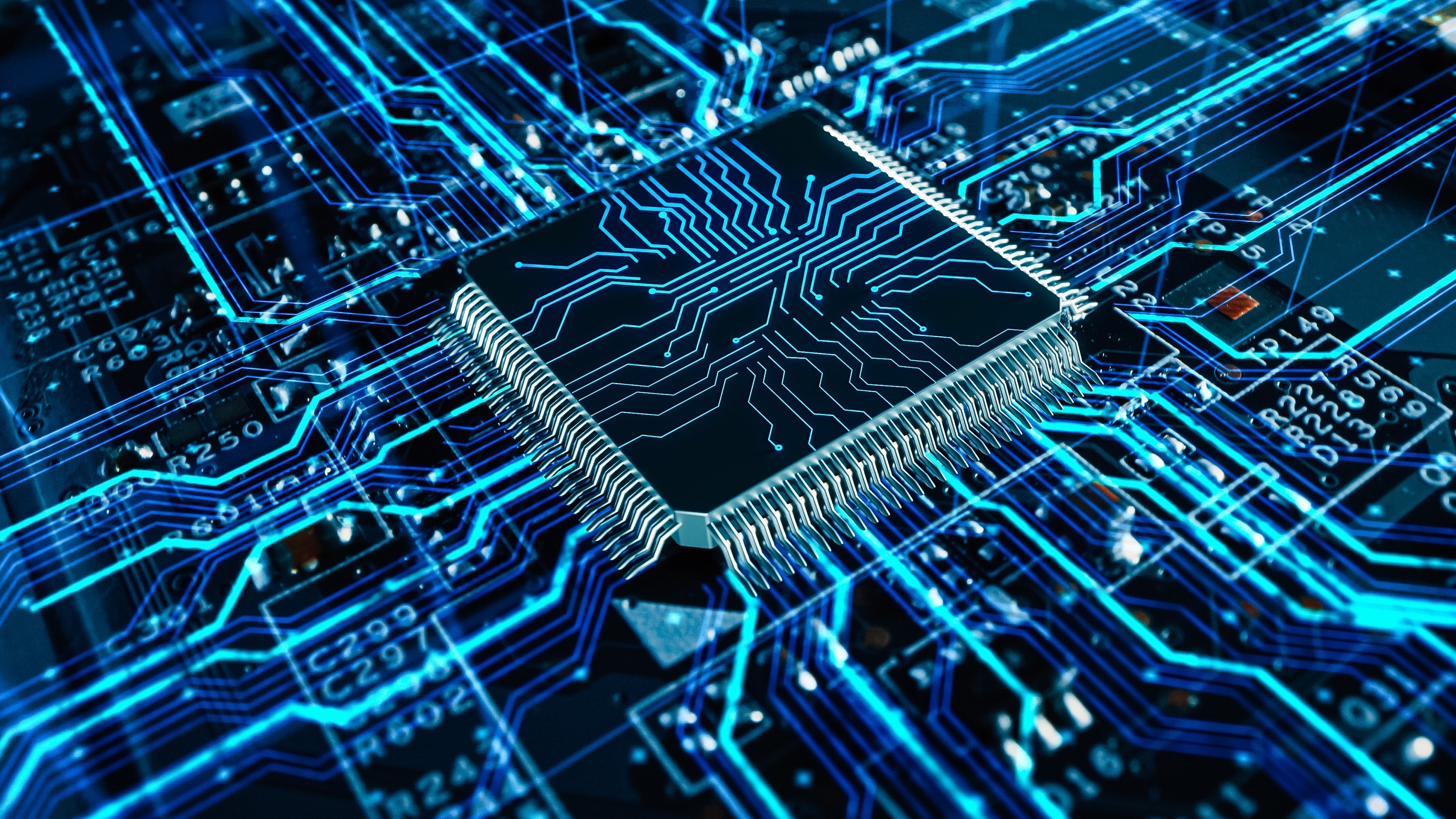
Our Publications
Our lab is at the forefront of cutting-edge AI research, covering a wide range of promising topics including AI chips/systems, compute-in-memory, electronic design automation, computer vision, vision-language, tiny machine learning, co-design, large foundational models, and medical image analysis.
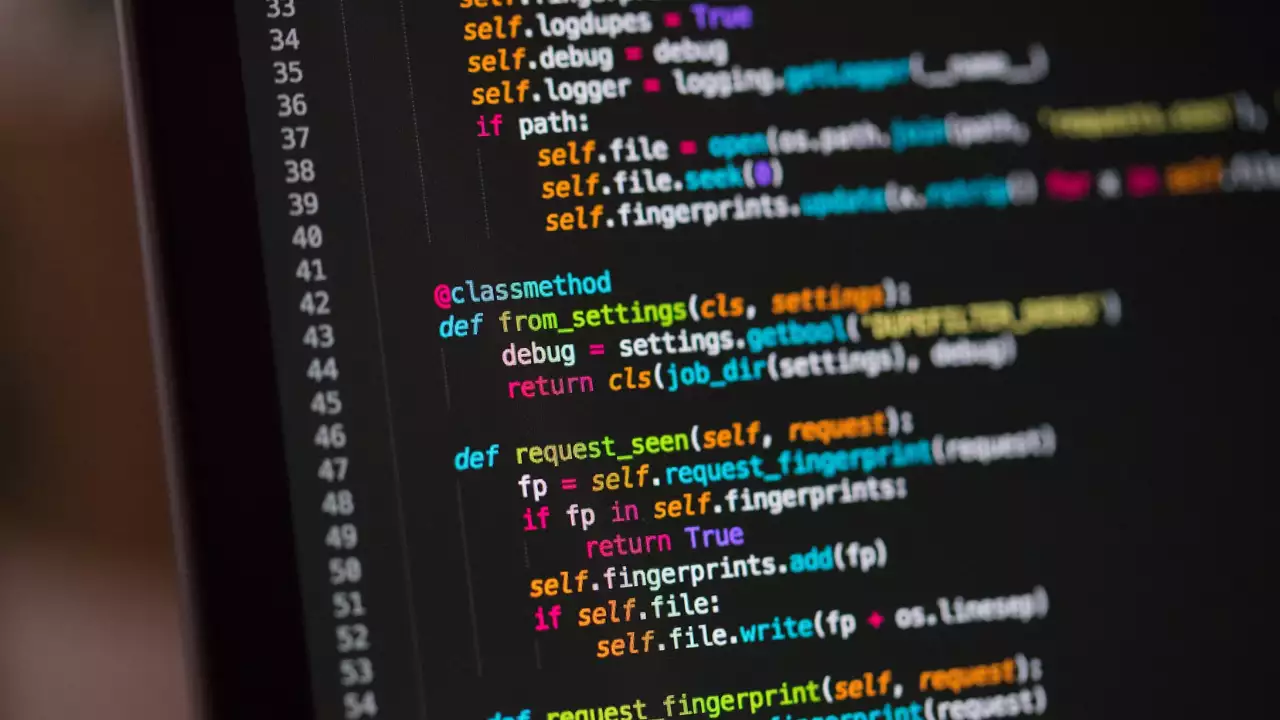
Our Research
Our research aims to make advanced AI technologies more effective and efficient, enabling everyone to enjoy the convenience and pleasure brought by these transformative technologies that will shape the future.